Climate change is arguably the most pressing issue of our time. Rising temperatures, extreme weather events, and diminishing natural resources call for urgent solutions. While many associate the fight against climate change with policy changes or renewable energy, technology has stepped in as an unlikely hero. Among the various advancements, machine learning (ML) has emerged as a game-changer, offering unprecedented tools to tackle the climate crisis.
In this article, we’ll explore how machine learning is transforming the way we combat climate change, its practical applications, and the potential it holds for a more sustainable future.
What is Machine Learning?
Before diving into its applications, let’s break down what machine learning is. Machine learning is a branch of artificial intelligence (AI) that enables computers to learn and improve from data without being explicitly programmed. Through algorithms and vast amounts of data, ML systems can identify patterns, make predictions, and optimize solutions.
This technology is uniquely positioned to address the complexities of climate change, which often involve analyzing massive datasets, modeling intricate systems, and identifying actionable insights.
Why Machine Learning is Vital in the Fight Against Climate Change
1. Climate Change is a Data Problem
The climate crisis involves analyzing enormous datasets, from atmospheric readings to ocean temperatures. Humans alone can’t process this level of complexity. Machine learning excels at managing, analyzing, and extracting valuable insights from such data.
2. Predictive Power
ML can predict climate trends and extreme weather events with greater accuracy, giving policymakers and organizations the information needed to act proactively.
3. Optimization for Sustainability
Machine learning enables better resource management by optimizing systems like energy grids, agriculture, and transportation. These optimizations reduce waste and carbon emissions.
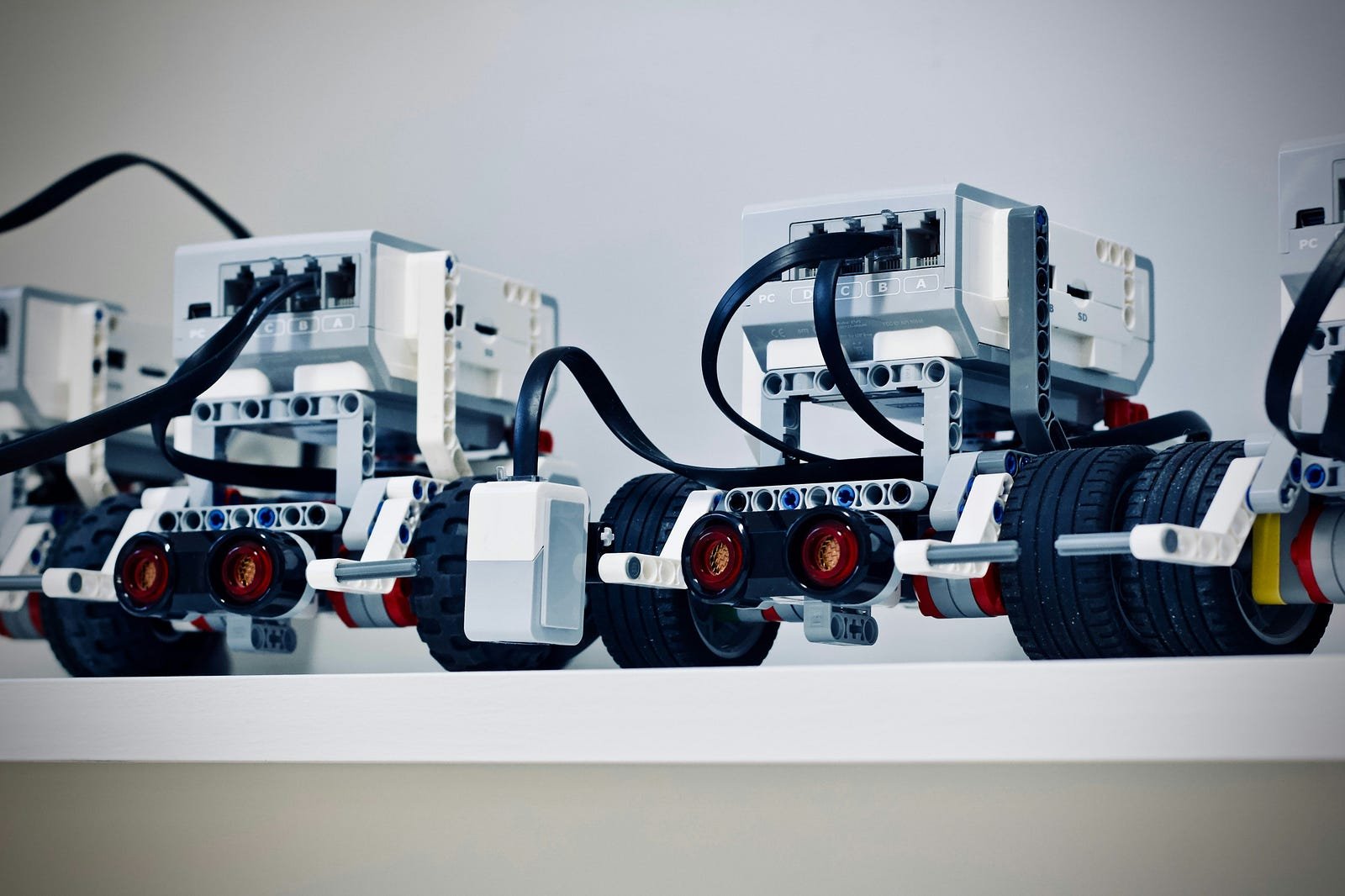
Practical Applications of Machine Learning in Combating Climate Change
1. Monitoring and Predicting Climate Change
Machine learning is revolutionizing climate monitoring and prediction.
- Enhanced Climate Models: Traditional climate models are limited in their ability to process dynamic systems. ML-based models can simulate complex climate interactions more accurately.
- Extreme Weather Prediction: ML algorithms analyze satellite data and historical weather patterns to forecast hurricanes, floods, and heatwaves earlier and with greater precision.
Real-World Example
Google’s AI research team uses ML to predict the environmental impact of energy systems, allowing more sustainable planning.
2. Renewable Energy Optimization
Renewable energy sources like solar and wind are vital for reducing greenhouse gas emissions. However, their intermittent nature poses challenges. Machine learning is addressing these issues.
- Energy Storage: ML optimizes battery storage, ensuring energy is available even when the sun isn’t shining or the wind isn’t blowing.
- Energy Forecasting: ML algorithms predict energy demand and production, enabling more efficient integration of renewable energy into power grids.
Real-World Example
IBM’s Deep Thunder project uses ML to optimize wind farm performance, boosting energy output while minimizing costs.
3. Reducing Carbon Emissions
Machine learning plays a crucial role in cutting emissions across various industries.
- Smart Transportation Systems: ML-powered navigation apps like Google Maps suggest eco-friendly routes, reducing fuel consumption. Autonomous vehicles, driven by ML algorithms, optimize fuel efficiency.
- Carbon Capture: ML improves the efficiency of carbon capture and storage (CCS) technologies by identifying the best locations and conditions for storing CO2.
Real-World Example
Microsoft’s Project Carbon AI uses ML to monitor and optimize carbon removal projects, ensuring maximum effectiveness.
4. Sustainable Agriculture
Agriculture contributes significantly to greenhouse gas emissions. Machine learning is transforming farming practices to make them more sustainable.
- Precision Agriculture: ML systems analyze soil health, weather patterns, and crop conditions to recommend optimal planting, irrigation, and harvesting strategies.
- Reducing Food Waste: ML algorithms in supply chain management predict demand more accurately, reducing overproduction and food spoilage.
Real-World Example
John Deere uses ML to guide autonomous tractors, improving planting efficiency while minimizing fuel use and soil degradation.
5. Forest and Ocean Conservation
Forests and oceans are critical for carbon sequestration, but they face threats from deforestation and pollution. Machine learning aids in their protection.
- Deforestation Monitoring: Satellite images analyzed by ML algorithms detect illegal logging activities in real time.
- Marine Conservation: ML identifies areas of ocean pollution, tracks marine species, and predicts the impact of climate change on coral reefs.
Real-World Example
Rainforest Connection uses ML-powered sensors to monitor illegal logging in rainforests, alerting authorities to intervene quickly.
6. Urban Planning and Smart Cities
Urban areas contribute a significant portion of global emissions. Machine learning helps design smarter, greener cities.
- Energy-Efficient Buildings: ML analyzes building data to optimize energy use in real time, reducing waste.
- Traffic Management: ML-powered systems reduce congestion, minimizing emissions from idling vehicles.
Real-World Example
Sidewalk Labs, a subsidiary of Alphabet, uses ML to design sustainable urban infrastructure.
The Challenges of Using Machine Learning for Climate Action
1. Energy Consumption of ML Models
Ironically, training large ML models requires significant computational power, which can have a high carbon footprint. Efforts are underway to make ML more energy-efficient.
2. Data Accessibility
ML relies on vast amounts of data, which isn’t always readily available or standardized, especially in developing regions.
3. Financial and Technological Barriers
Implementing ML solutions requires funding and expertise, which may not be accessible to all governments and organizations.
Future Innovations in Machine Learning for Climate Change
The potential of machine learning in combating climate change is just beginning to unfold. Here are some exciting possibilities on the horizon:
1. Climate Risk Modeling
ML will continue improving models that predict the economic and social impacts of climate change, guiding better policy decisions.
2. Carbon Market Optimization
Machine learning can enhance carbon trading markets by tracking and verifying carbon credits with greater accuracy.
3. Enhanced Biodiversity Monitoring
AI-powered drones and sensors, guided by ML algorithms, will monitor ecosystems more comprehensively to track biodiversity and detect environmental changes.
Ethical Considerations in Using Machine Learning for Climate Action
1. Inclusivity
Ensuring that ML solutions benefit all regions, particularly vulnerable populations in developing countries, is crucial.
2. Privacy Concerns
Data collection for ML models must respect privacy and avoid misuse of personal or community data.
3. Transparency
ML systems must be transparent and interpretable to build trust among stakeholders and ensure accountability.
How You Can Support ML-Driven Climate Action
1. Advocate for Funding
Encourage governments and private organizations to invest in ML research and development for climate solutions.
2. Support Green Tech Startups
Many startups are leveraging ML to tackle environmental issues. Supporting these innovators accelerates the adoption of sustainable technologies.
3. Stay Informed
Educate yourself and others about the potential of machine learning in combating climate change to build awareness and drive collective action.
Machine learning offers a beacon of hope in the fight against climate change. From optimizing renewable energy to protecting ecosystems and reducing emissions, its applications are vast and impactful. However, leveraging ML responsibly and inclusively is critical to its success.
As we navigate the climate crisis, embracing innovative technologies like machine learning will be key to creating a sustainable future. By investing in this tech revolution, we can transform challenges into opportunities and ensure a healthier planet for generations to come.